Coming up Next are all Liabilities of Subsidiary Classifiers with the Exception of
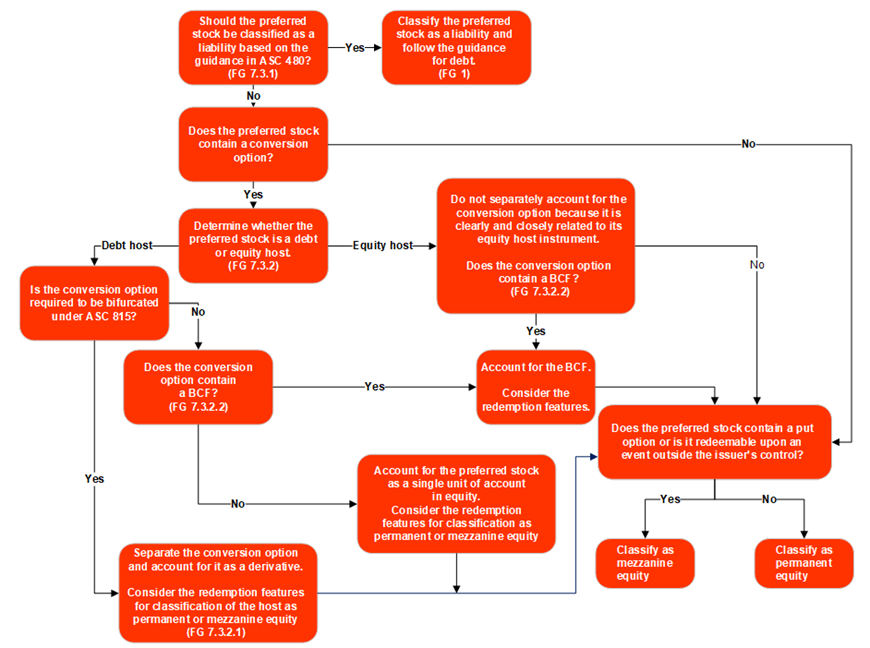
In the intricate world of classification models, subsidiary classifiers play a crucial role in enhancing the accuracy and performance of machine learning systems. These classifiers are designed to complement the primary model, contributing to a more nuanced and sophisticated understanding of data. However, not all that glitters is gold; within this framework, liabilities come into play. In this article, we will delve into the liabilities associated with subsidiary classifiers, highlighting exceptions that distinguish them from their primary counterparts.
Defining Subsidiary Classifiers:
Before we explore the liabilities, let’s establish a clear understanding of subsidiary classifiers. In the realm of machine learning, a subsidiary classifier is an additional layer or model integrated into a primary classification system to address specific challenges or nuances within the data. These classifiers can enhance the primary model’s performance by focusing on particular subsets or patterns that may be challenging for the primary model to discern accurately.
Common Subsidiary Classifier Liabilities:
- Overfitting: One of the primary liabilities associated with subsidiary classifiers is overfitting. As these classifiers are often trained to address specific subsets of data, there is a risk of over-optimization for those particular patterns. This can result in the subsidiary classifier performing exceptionally well on the training data but failing to generalize effectively to new, unseen data.
- Increased Complexity: Introducing subsidiary classifiers can significantly increase the overall complexity of the classification model. This complexity may lead to longer training times, increased computational requirements, and challenges in model interpretability. Balancing the benefits of enhanced accuracy with the drawbacks of increased complexity is a delicate task.
- Data Imbalance: Subsidiary classifiers are prone to encountering issues related to data imbalance, especially when dealing with specific subsets that may have limited representation in the overall dataset. This can lead to biased predictions, where the model is more inclined to favor the majority class and struggle with minority classes.
- Integration Challenges: Integrating subsidiary classifiers seamlessly into a primary classification system can be challenging. Ensuring that the models work in harmony, share information effectively, and contribute positively to the overall prediction requires careful design and testing.
- Maintenance Overhead: Managing and maintaining subsidiary classifiers adds an extra layer of responsibility. Updates to the primary model or changes in data distribution may necessitate retraining and fine-tuning the subsidiary classifiers to maintain optimal performance. This ongoing maintenance can be resource-intensive.
Exceptions to the Rule:
While subsidiary classifiers come with their set of liabilities, certain exceptions distinguish them from primary classifiers. These exceptions contribute to the uniqueness of subsidiary classifiers and their specialized roles within machine learning systems.
- Niche Expertise: Subsidiary classifiers often exhibit a niche expertise in handling specific types of data or patterns. Unlike primary classifiers, which aim for a broad understanding of the entire dataset, subsidiary classifiers excel in their domain of specialization. This can be particularly advantageous when dealing with complex datasets with diverse characteristics.
- Adaptability to Specialized Tasks: Subsidiary classifiers are adept at handling specialized tasks that may be challenging for a single, generic classifier. Their ability to focus on particular subsets allows them to excel in scenarios where nuanced insights are crucial, such as fraud detection, anomaly identification, or sentiment analysis within specific contexts.
- Ensemble Learning Benefits: Subsidiary classifiers contribute to ensemble learning strategies, where the combination of multiple models leads to superior predictive performance. Ensemble methods leverage the strengths of individual classifiers while mitigating their weaknesses, resulting in a more robust and accurate overall model.
- Improved Feature Representation: By concentrating on specific subsets, subsidiary classifiers can enhance feature representation for particular patterns in the data. This can lead to a more nuanced understanding of complex relationships and improve the overall interpretability of the model.
Conclusion:
In the intricate landscape of machine learning, subsidiary classifiers emerge as valuable tools for addressing the inherent challenges posed by diverse and complex datasets. While their liabilities, such as overfitting and increased complexity, are acknowledged, their exceptions set them apart, offering specialized expertise and adaptability to handle tasks that primary classifiers may find challenging.
Understanding the delicate balance between the advantages and drawbacks of subsidiary classifiers is essential for data scientists and machine learning practitioners. As technology advances, refining the design, integration, and maintenance of subsidiary classifiers will be crucial for harnessing their full potential and unlocking new frontiers in the realm of classification models.